What do we need to know to make this project work and how will we know if it worked? These are two fundamental questions we ask ourselves as practitioners. To answer these questions we rely on research. Research evidence is one of the most powerful tools we have in our development toolbox to specify problems, identify root causes, and use our resources strategically to encourage meaningful change and improvements in people's lives. However, researchers, like all humans, are prone to bias — and if left unchecked, that bias can make research evidence less reliable. As the commissioners of this research we are often guilty of contributing to bias with some of the choices we make. However, by understanding common types of bias and how to identify them, we have the opportunity to use well-tested strategies and tools to protect against bias and create the best-informed programs.
Bias refers to systematic faulty judgments we make while observing or affecting the world around us. We often have to make quick judgments, and do this by coming up with generalized rules to make those decisions easier. These generalized rules are adaptive tools which can help us simplify the world around us, but they won’t always serve our interests.
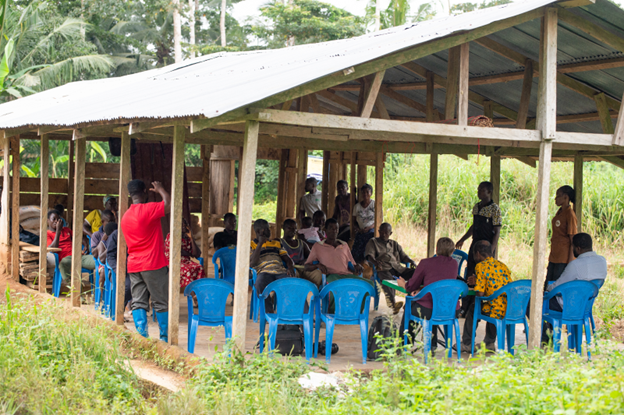 An impact evaluation team conducts a scoping visit in the village of Meteameba near Asankragowa, Ghana, photo: Cloudburst Group.
Bias can come into play with many of the decisions we make when commissioning or conducting research and interpreting evidence, and can impact the researcher, the research participant, the person using the research — and sometimes all three!
In this Learning Digest, we’ll explore types of bias and how to counter them:
- Choosing the Right Team: Researcher Bias
- Knowing Who You’re Talking to: Participant Bias
- Am I the Problem?: Funder/Client Bias
Please make use of DRG Evidence and Learning Team resources! (See text box at the end.)
Choosing the Right Team: Researcher Bias
We all know that the right team makes all the difference. Researchers can unknowingly bias the results of their studies in a number of different ways. Choosing the right research team with potential areas of bias in mind is critical to the success of the research. Let us touch on three types of potential bias by researchers: selection bias, methodological bias, and analytical bias.
Selection bias: Since it would be prohibitively expensive and time-consuming to ask all potential recipients of an aid program about how it helped them, we instead try to gather a representative sample that can reflect the experiences of the total population. However, if the group we choose doesn’t represent the larger population we are trying to draw conclusions about, we will get biased results. Sometimes this bias arises when the people who participate are chosen based on availability or interest in participation in a study, or due to an underlying gender bias that influences both the selection of participants in research and perceptions about individuals’ capacity to do quality research. When our sample does not include diverse perspectives (or contexts), it limits the external validity and generalizability of the findings. In another example of selection bias, research criticizing International Monetary Fund (IMF) stabilization programs often cites findings that countries tend to decline economically as a result of IMF stabilization programs. But do they? Other researchers explored whether economic declines were because of IMF programs or because the only countries to get involved in IMF programs were already on the decline. When the researchers compared economic decline between IMF program recipients and matching countries that were not IMF recipients, they found that IMF recipients were not seeing any additional economic decline.
Methodological bias: Many of us select research teams based on prior experience doing similar types of research. These researchers have experience collecting data and evidence, and have preferred approaches that they are more familiar and comfortable with. But sometimes, comfortable methodologies may not get us the information we need. Reconsidering our methods and epistemologies, and bringing in alternative methods, may result in producing knowledge that engages, empowers, and advances equity for the target population. For example, if we want to test a program’s effectiveness, we are better off using a quantitative randomized controlled trial in a robust impact evaluation than a performance evaluation, or in other cases quasi-experimental designs may be a better fit when experimental designs are not always feasible or ethical.
Bias can also be reflected in some of the specific methods a researcher chooses to use. For example, an agriculture program in Mexico using SMS-delivered information noted that the technology was simultaneously inaccessible for those who were mobile-illiterate and too antiquated for smartphone-literate audiences. Being tied to a particular methodology can limit our effectiveness. Additionally, while we may be used to having outside researchers conduct interviews, we should be aware that it’s not enough to just speak a local language, but it’s also important to “speak” a local culture. For example, research in African countries needs culturally informed language for questions like what constitutes a household. Can we ask about their household, or do we need to ask whether they are eating from the same pot or living under the same roof?
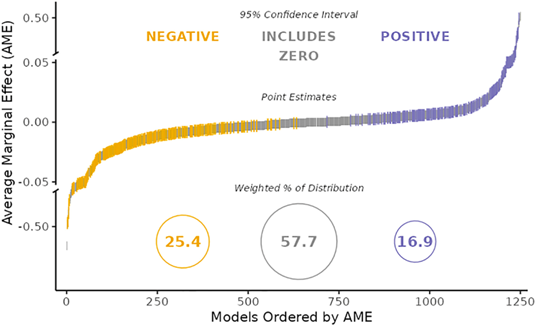 Analytical Bias: Broad variation in the findings from 73 teams testing the same hypothesis with the same data.
Analytical bias: Suppose you conducted a great study with all the right people. Now you have a lot of data, and you need to figure out how to interpret it. How do you understand all the information you gathered in a way that best represents the world? What do you compare? Do you include all of the data? What kinds of analyses are right for the information you gathered? The answers to these questions are not as obvious as we might think, allowing for analytical bias to creep in. For example, the graph above demonstrates how researchers took a dataset on the effect of immigration on support for social welfare policies and shared it with 73 teams of researchers and asked them to analyze the data. The teams reported a wide range of interpretations of the data, with almost 58 percent reporting no meaningful relationship, over 25 percent reporting that immigration reduces support for social welfare policies, and nearly 17 percent finding a positive relationship. The role of analytical decisions in changing the interpretation of data has also been found with the effect of scientists’ gender and status on how talkative they were in meetings, which has implications for leadership and decision making.
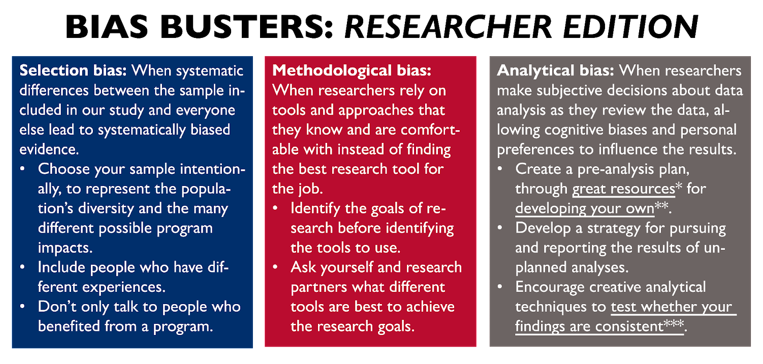 Researcher bias can significantly impact our findings but tools like a pre-analysis plan can help us avoid these pitfalls. While developing your own pre-analysis plan is a critical early step, don’t forget to also test whether your findings are consistent and check out this practical guidebook with tools to collect, compile, analyze, and disseminate disaggregated data.
Knowing Who You’re Talking to: Participant Bias
We never collect data in a vacuum. There is almost always someone at the other end, answering our questions and responding to our surveys. A mistake that some people make is to forget that the people they will ask questions of are questioning, wondering, and judging what you want to hear and how they should respond. Participant bias is a second key area of potential bias in our evidence and findings, and here we will touch on three types: self-selection bias, acquiescence bias, and social desirability bias.
Self-selection bias: Our participants can also be a source of bias — not from wanting to undermine our research, but from wanting to help it. People who feel they have more to gain from the research will want to participate, and might suggest other people who think similarly for the researchers to contact. Their associations with the U.S. government and its values, their desire for funding, or other opportunities might all change whether or not they choose to participate, which can disrupt the balance of respondents and the results as a whole. In some countries the presence of a foreigner accompanied by a local could be interpreted negatively, with one or both assumed to be intelligence agents for governments.
Acquiescence bias: Does how a question is asked affect the answer you might get? The British political comedy series, Yes, Prime Minister, would say that it does. Acquiescence bias refers to some people’s tendency to agree to questions or statements, even when they don’t necessarily believe them. One study suggests that this bias can lead to up to a 50 percent increase in people agreeing with conspiracy theories and political misconceptions. In another study looking at patient satisfaction, researchers found that acquiescence bias changed the interpretation of patient satisfaction data in Nigeria. When the same questions were asked with a negative frame rather than a positive frame, the percentage of patients who were satisfied with their experience declined up to 19 percent.
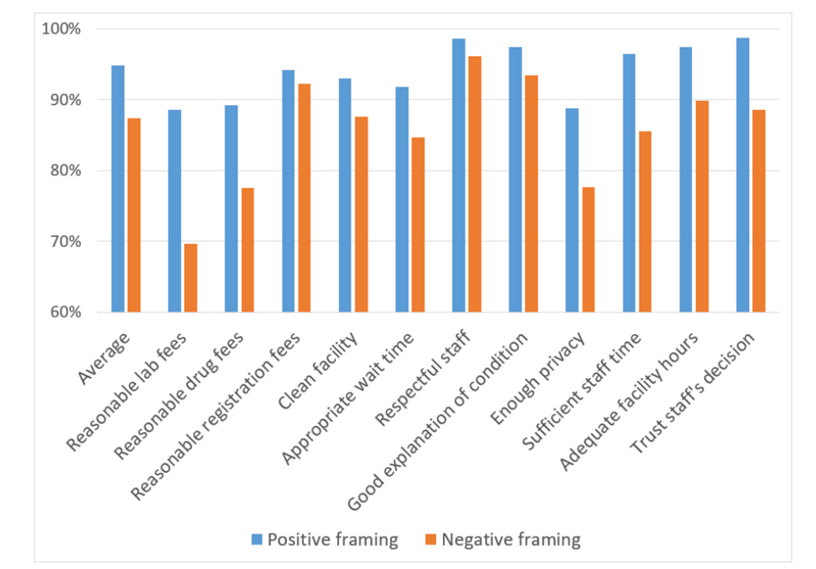 Patient satisfaction is easily manipulated by framing questions toward or away from acquiescence. Percentage of patients who respond “I agree” to a statement about the quality of care that they received at a primary healthcare facility. Based on a sample of 2,222 patients across six Nigerian states.
Social desirability bias: The self-selection bias is closely connected to social desirability bias. People will often choose responses based on what they believe the questioner wants to hear, or based on other social influences of expected responses. For example, research indicates that participants will sometimes present untrue narratives and tropes in interviews, with some participants sharing different information after a recording device is turned off. The likelihood of getting responses affected by social desirability are more likely with sensitive topics or sensitive settings for the interview. Research into vote-buying in Nicaragua noted that while only two percent of respondents agreed that they had been offered gifts or money in exchange for their votes when they were asked directly within a survey, 24 percent agreed when they were asked indirectly.
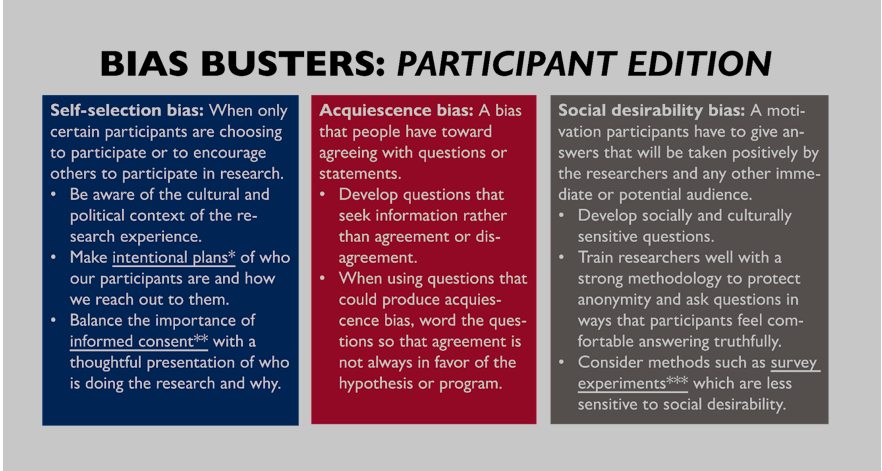 Many USAID research projects fall into the participant bias trap. To avoid this bias, consider developing intentional plans for outreach. Don’t forget the importance of informed consent (see this resource). And always remember these 10 Things to Know About Survey Experiments.
Am I the Problem? Funder/Client Bias
Sometimes we forget that as the funders or clients of research projects we are also actors whose presence and requests can contribute to bias if we are not careful. For example, the interviewees or participants who are benefiting directly from the programs that we are conducting, and the researchers who are working directly for us, may want to provide the results they think we want to see. Here we will focus on two types of bias which can come from the funders or clients of our research: confirmation bias and publication/dissemination bias.
Confirmation bias: This is a general cognitive bias where we tend to look for information that confirms what we already think to be true, rather than trying to falsify our beliefs. Funders and clients are not immune from being biased in favor of their pre-existing beliefs. Research with development practitioners from the World Bank and the Department for International Development in the United Kingdom found that people were more likely to correctly recall the findings of a report when it aligned with their personal worldviews. When we are in the role of client or funder, we also have a privileged position from which to make recommendations about samples to include in our studies and products; how to present findings, appropriate language, and preferred methodology; and other decisions. While we often have important insights that can enrich a research project, we have to be careful that client or funder insights do not cross the line into biasing the results of our research. For example, a study found that increased political diversity improves social psychological science by reducing the impact of confirmation bias, and by empowering dissenting minorities to improve the quality of the majority’s thinking.
Publication/dissemination bias: Even when we develop a completely unbiased research project and product, there are still some other decisions we have to make that can bias our continued work. One example is publication bias, where studies that do not find a statistically significant finding are left unpublished, which can bias a reading of the literature in the field. After all, if many studies showing no effect of behavioral nudging are left unpublished, then we may be unable to conclude whether or not behavioral nudges really do work and when. Many academic disciplines have been noting this problem for over 40 years, and some have adopted statistical techniques to estimate publication bias and what that means for the findings in the published literature. Sometimes our decision of whether or how to disseminate or publish the results of our research differs based on what we think of the findings, even if not intentionally. And what we say and what we don’t say about what works (and who gets to say it) can influence the programmatic decisions that other development professionals take. Therefore, when feasible (and without putting participants in harm’s way), findings should be shared with both the research community and the target population.
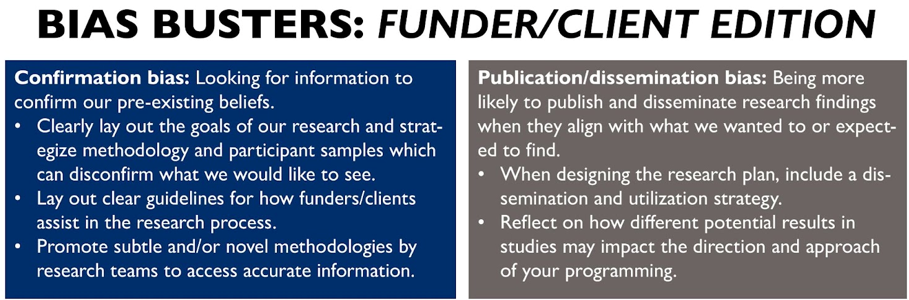 As the funder/client we are often guilty of unduly influencing the research we commission, but the guidelines above can help us avoid falling into funder/client bias.
What does this all mean for evidence?
As we learn from Spiderman, with great power comes great responsibility. Evidence has great power to enable us to work with limited resources in difficult spaces and empower people to create positive change. Good evidence can tell us where to invest, where to adapt, and where to collaborate, and with so much power, we should make sure that we use it responsibly. By being aware of the biases that can affect our work and change the conclusions of our evidence, we can take steps to avoid them by being thoughtful and engaged in the research process.
Further Assistance
For further assistance on how to identify and address bias in your work, contact the DRG Bureau’s Evidence and Learning Team at drg.el@usaid.gov.
Recent DRG Learning Events
E&L Talk Series: Information Integrity in the Global South: Detection, Consequences, and How to Counter Falsehoods – Information integrity is a growing concern among the public and policymakers. Around the world, many news outlets and governmental agencies are sounding the alarm regarding the political and societal threats posed by a lack of information integrity. Yet significant findings from academic studies suggest that the effects of false information are limited, and concerns about "post-truth" politics are possibly exaggerated. On December 7, Dr. Natália S. Bueno joined the DRG Bureau to share her reflections on this puzzling mismatch by discussing what we know about the prevalence of false information in political discourse from political leaders and how we detect false information using statistical techniques. Using evidence from Brazil, Dr. Bueno’s research focuses on the consequences of false information shared by political leaders, and what we can do to counter it. Overall, the studies reveal that false information has strikingly heterogeneous consequences depending on the audience receiving it and the type of action it may induce, and similarly, the effectiveness of tools to combat false information is contingent upon the content of the false information.
Use Our Resources!
Welcome to the DRG Learning Digest, a newsletter to keep you informed of the latest learning, evaluation, and research in the Democracy, Human Rights, and Governance (DRG) sector. Views expressed in the external (non-USAID) publications linked in this Digest do not necessarily represent the views of the United States Agency for International Development or the United States Government.
Want the latest DRG evidence, technical guidance, events and more? Check out the new https://www.drglinks.org/.
Don't forget to check out our DRG Learning Menu of Services! (Link only accessible to USAID personnel.) The Menu provides information on the learning products and services the Evidence and Learning Team offers to help you fulfill your DRG learning needs. We want to help you adopt learning approaches that emphasize best fit and quality.
The Evidence and Learning Team is also excited to share our DRG Learning, Evidence, and Analysis Platform (LEAP) with you. This Platform contains an inventory of programmatic approaches, evidence gap maps, the DRG Learning Harvest, and inventories of indicators and country data portraits - all of which can be very useful in DRG activity design, implementation, evaluation, and adaptation. Some of these resources are still being built, so check back frequently to see what has been newly added.
The DRG Learning Harvest on LEAP is a searchable database of DRG learning products, including summaries of key findings and recommendations, drop-down menus to easily find documents related to a particular country or program area, and links to the full reports on the DEC.
Our friends at the Varieties of Democracy (V-Dem) Institute are also seeking to expand their research partnership with USAID on the complex nature of democracy by inviting research questions from you for V-Dem to work on. If there's a DRG technical question you've been wondering about, please email the Evidence and Learning Team at drg.el@usaid.gov.
We welcome your feedback on this newsletter and on our efforts to promote the accessibility, dissemination, and utilization of DRG evidence and research. Please visit the DRG Bureau's website for additional information or contact us at DRG.EL@usaid.gov.
|